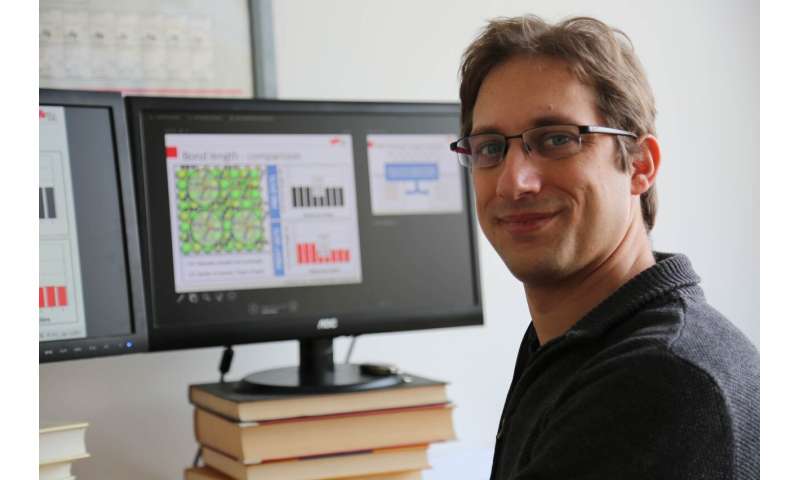
Oliver Hofmann and his research group at the Institute of Solid State Physics at TU Graz are working on the optimization of modern electronics. A key role in their research is played by interface properties of hybrid materials consisting of organic and inorganic components, which are used, for example, in OLED displays or organic solar cells. The team simulates these interface properties with machine-learning-based methods. The results are used in the development of new materials to improve the efficiency of electronic components.
Long-range charge transfer as a subject of investigation
The researchers have now taken up the phenomenon of long-range charge transfer. A transfer of electrons from one material to another already occurs in the switched-off state if there are energetically more favorable states for the electrons in the neighboring material. This raises the fundamental question of how far this transfer of electrons can extend in organic material, i.e. how many layers it encompasses. Many studies report that for organic-inorganic interfaces this effect is limited to the first layer, i.e. the layer where the (organic) molecules are in direct contact with the (inorganic) metal surface.
On the other hand, some reports assume that the effect also extends over longer distances, to the second layer or beyond. "If this is the case, the effect could be used to reduce the electrical resistance of the hybrid material, making it more energy efficient," says Hofmann, explaining why it is so interesting.
New examination method combines two machine learning methods
In order to demonstrate long-range charge transport in organic-inorganic interfaces, the researchers used the new machine learning methods SAMPLE and BOSS to investigate a copper-tetracyanoethylene interface (TCNE/Cu(111)), "since there are particularly strong experimental data available here that indicate long-range charge transport," said Hofmann. There is no clear theory why some systems show this effect. Hofmann and his team wanted to "solve this mystery in order to create a basis for how to produce materials with the same property."
By combining both methods, the researchers were able to identify over two million potential interface structures for the TCNE-Cu interfaces and predict the behavior of the molecules under various experimental conditions. Surprisingly, the results showed that there is no long-range charge transfer, but instead the molecules in the system change their structure.
Molecules change their atomic arrangement
When molecules are applied, usually they retain their general arrangement and pack more closely until, at a certain density, they finally start growing the second layer. In the TCNE/Cu(111) system, however, the adsorbed molecules change from the original lying position to a standing one after a certain amount has been deposited. They thus straighten up in order to be able to crowd even closer together. "However, standing molecules have a completely different charge transfer than lying molecules. The structural transformation is difficult to detect experimentally, but the measurement results are similar to those of long-range charge transport," explains Hofmann.
The investigations refute the hypothesis of long-range charge transfer. The use of the combined machine learning methods SAMPLE and BOSS is intended to support future experiments in material development in such a way that such misinterpretations no longer occur. By taking a deeper look into the physical processes, the new processes help to ensure that materials are no longer designed to chase an effect that does not exist in this form. Hofmann underlines the advantage of the new method: "Thanks to the two methods, millions of different structures can be simulated in the future."
The TU Graz researchers recently published details of the study in Advanced Science.
Explore further
Citation: Machine learning methods provide new insights into organic-inorganic interfaces (2020, August 4) retrieved 4 August 2020 from https://ift.tt/2Xsqkr2
This document is subject to copyright. Apart from any fair dealing for the purpose of private study or research, no part may be reproduced without the written permission. The content is provided for information purposes only.
"interface" - Google News
August 05, 2020 at 01:03AM
https://ift.tt/2Xsqkr2
Machine learning methods provide new insights into organic-inorganic interfaces - Phys.org
"interface" - Google News
https://ift.tt/2z6joXy
https://ift.tt/2KUD1V2
Bagikan Berita Ini
0 Response to "Machine learning methods provide new insights into organic-inorganic interfaces - Phys.org"
Post a Comment