Abstract
Neuropsychiatric disorders such as major depression are a leading cause of disability worldwide with standard treatments, including psychotherapy or medication, failing many patients. Deep brain stimulation holds great potential as an alternative therapy for treatment-resistant cases; however, improving the efficacy of stimulation therapy for neuropsychiatric disorders is hindered by the complexity as well as inter-individual and intra-individual variability in symptom manifestations, neural representations and response to therapy. These challenges motivate the development of brain–computer interfaces (BCIs) that can decode the symptom state of a patient from brain activity as feedback to personalize the stimulation therapy in closed loop. Here we review progress on developing BCIs for neuropsychiatric care, focusing on neural biomarkers for decoding symptom states, stimulation site selection and closed-loop stimulation strategies. Moreover, we highlight promising data-driven machine learning and system design approaches and provide a roadmap for realizing these BCIs. Finally, we review current limitations, discuss extensions to other treatment modalities and outline the required scientific and technological advances. These advances can enable next-generation BCIs that provide an alternative therapy for treatment-resistant neuropsychiatric disorders.
This is a preview of subscription content, access via your institution
Access options
Subscribe to this journal
Receive 12 digital issues and online access to articles
$99.00 per year
only $8.25 per issue
Buy this article
- Purchase on Springer Link
- Instant access to full article PDF
Prices may be subject to local taxes which are calculated during checkout
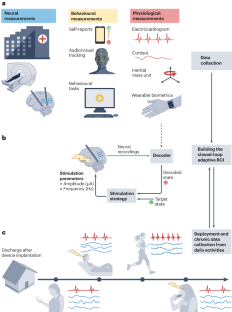
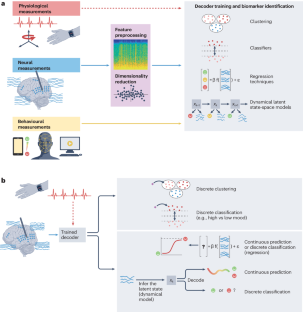
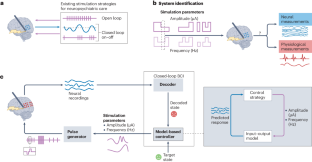
References
-
Ferrari, A. Global, regional, and national burden of 12 mental disorders in 204 countries and territories, 1990–2019: a systematic analysis for the Global Burden of Disease Study 2019. Lancet Psychiatry 9, 137–150 (2022).
-
Whiteford, H. A. et al. Global burden of disease attributable to mental and substance use disorders: findings from the Global Burden of Disease Study 2010. Lancet 382, 1575–1586 (2013).
-
Rush, A. J. et al. Acute and longer-term outcomes in depressed outpatients requiring one or several treatment steps: a STAR*D report. Am. J. Psychiatry 163, 1905–1917 (2006).
-
Mrazek, D. A., Hornberger, J. C., Altar, C. A. & Degtiar, I. A review of the clinical, economic, and societal burden of treatment-resistant depression: 1996–2013. Psychiatr. Serv. 65, 977–987 (2014).
-
Olchanski, N. et al. The economic burden of treatment-resistant depression. Clin. Ther. 35, 512–522 (2013).
-
Greenberg, P. E., Fournier, A. A., Sisitsky, T., Pike, C. T. & Kessler, R. C. The economic burden of adults with major depressive disorder in the United States (2005 and 2010). J. Clin. Psychiatry 76, 155–162 (2015).
-
Nuttin, B., Cosyns, P., Demeulemeester, H., Gybels, J. & Meyerson, B. Electrical stimulation in anterior limbs of internal capsules in patients with obsessive–compulsive disorder. Lancet 354, 1526 (1999).
-
Fontaine, D. et al. Effect of subthalamic nucleus stimulation on obsessive–compulsive disorder in a patient with Parkinson disease: case report. J. Neurosurg. 100, 1084–1086 (2004).
-
Mayberg, H. S. et al. Deep brain stimulation for treatment-resistant depression. Neuron 45, 651–660 (2005).
-
Greenberg, B. D. et al. Three-year outcomes in deep brain stimulation for highly resistant obsessive–compulsive disorder. Neuropsychopharmacology 31, 2384–2393 (2006).
-
Schlaepfer, T. E. et al. Deep brain stimulation to reward circuitry alleviates anhedonia in refractory major depression. Neuropsychopharmacology 33, 368–377 (2007).
-
Lozano, A. M. et al. Subcallosal cingulate gyrus deep brain stimulation for treatment-resistant depression. Biol. Psychiatry 64, 461–467 (2008).
-
Mallet, L. et al. Subthalamic nucleus stimulation in severe obsessive–compulsive disorder. N. Engl. J. Med. 359, 2121–2134 (2008).
-
Malone, D. A. et al. Deep brain stimulation of the ventral capsule/ventral striatum for treatment-resistant depression. Biol. Psychiatry 65, 267–275 (2009).
-
Denys, D. et al. Deep brain stimulation of the nucleus accumbens for treatment-refractory obsessive–compulsive disorder. Arch. Gen. Psychiatry 67, 1061–1068 (2010).
-
Sartorius, A. et al. Remission of major depression under deep brain stimulation of the lateral habenula in a therapy-refractory patient. Biol. Psychiatry 67, e9–e11 (2010).
-
Schlaepfer, T. E., Bewernick, B. H., Kayser, S., Mädler, B. & Coenen, V. A. Rapid effects of deep brain stimulation for treatment-resistant major depression. Biol. Psychiatry 73, 1204–1212 (2013).
-
Luyten, L., Hendrickx, S., Raymaekers, S., Gabriëls, L. & Nuttin, B. Electrical stimulation in the bed nucleus of the stria terminalis alleviates severe obsessive–compulsive disorder. Mol. Psychiatry 21, 1272–1280 (2016).
-
Riva-Posse, P. et al. A connectomic approach for subcallosal cingulate deep brain stimulation surgery: prospective targeting in treatment-resistant depression. Mol. Psychiatry 23, 843–849 (2017).
-
Fenoy, A. J. et al. A longitudinal study on deep brain stimulation of the medial forebrain bundle for treatment-resistant depression. Transl. Psychiatry 8, 111 (2018).
-
Rao, V. R. et al. Direct electrical stimulation of lateral orbitofrontal cortex acutely improves mood in individuals with symptoms of depression. Curr. Biol. 28, 3893–3902.e4 (2018).
-
Crowell, A. L. et al. Long-term outcomes of subcallosal cingulate deep brain stimulation for treatment-resistant depression. Am. J. Psychiatry 176, 949–956 (2019).
-
Denys, D. et al. Efficacy of deep brain stimulation of the ventral anterior limb of the internal capsule for refractory obsessive–compulsive disorder: a clinical cohort of 70 patients. Am. J. Psychiatry 177, 265–271 (2020).
-
Graat, I. et al. Long-term outcome of deep brain stimulation of the ventral part of the anterior limb of the internal capsule in a cohort of 50 patients with treatment-refractory obsessive–compulsive disorder. Biol. Psychiatry 90, 714–720 (2021).
-
Menchón, J. M. et al. A prospective international multi-center study on safety and efficacy of deep brain stimulation for resistant obsessive–compulsive disorder. Mol. Psychiatry 26, 1234–1247 (2021).
-
Scangos, K. W. et al. Closed-loop neuromodulation in an individual with treatment-resistant depression. Nat. Med. 27, 1696–1700 (2021).
-
Shivacharan, R. S. et al. Pilot study of responsive nucleus accumbens deep brain stimulation for loss-of-control eating. Nat. Med. 28, 1791–1796 (2022).
-
Gill, J. L. et al. A pilot study of closed-loop neuromodulation for treatment-resistant post-traumatic stress disorder. Nat. Commun. 14, 2997 (2023).
-
Dougherty, D. D. et al. A randomized sham-controlled trial of deep brain stimulation of the ventral capsule/ventral striatum for chronic treatment-resistant depression. Biol. Psychiatry 78, 240–248 (2015).
-
Holtzheimer, P. E. et al. Subcallosal cingulate deep brain stimulation for treatment-resistant depression: a multisite, randomised, sham-controlled trial. Lancet Psychiatry 4, 839–849 (2017).
-
Widge, A. S. et al. Treating refractory mental illness with closed-loop brain stimulation: progress towards a patient-specific transdiagnostic approach. Exp. Neurol. 287, 461–472 (2017).
-
Provenza, N. R. et al. The case for adaptive neuromodulation to treat severe intractable mental disorders. Front. Neurosci. 13, 152 (2019).
-
Figee, M. et al. Deep brain stimulation for depression. Neurotherapeutics 19, 1229–1245 (2022).
-
Drevets, W. C. Neuroimaging and neuropathological studies of depression: implications for the cognitive-emotional features of mood disorders. Curr. Opin. Neurobiol. 11, 240–249 (2001).
-
Williams, L. M. Defining biotypes for depression and anxiety based on large-scale circuit dysfunction: a theoretical review of the evidence and future directions for clinical translation. Depress. Anxiety 34, 9–24 (2017).
-
Baldermann, J. C. et al. Connectomic deep brain stimulation for obsessive–compulsive disorder. Biol. Psychiatry 90, 678–688 (2021).
-
Li, N. et al. A unified connectomic target for deep brain stimulation in obsessive–compulsive disorder. Nat. Commun. 11, 3364 (2020).
-
Riva-Posse, P. et al. Defining critical white matter pathways mediating successful subcallosal cingulate deep brain stimulation for treatment-resistant depression. Biol. Psychiatry 76, 963–969 (2014).
-
Mulders, P. C., van Eijndhoven, P. F., Schene, A. H., Beckmann, C. F. & Tendolkar, I. Resting-state functional connectivity in major depressive disorder: a review. Neurosci. Biobehav. Rev. 56, 330–344 (2015).
-
Makris, N. et al. Variability and anatomical specificity of the orbitofrontothalamic fibers of passage in the ventral capsule/ventral striatum (VC/VS): precision care for patient-specific tractography-guided targeting of deep brain stimulation (DBS) in obsessive compulsive disorder (OCD). Brain Imaging Behav. 10, 1054–1067 (2016).
-
Drysdale, A. T. et al. Resting-state connectivity biomarkers define neurophysiological subtypes of depression. Nat. Med. 23, 28–38 (2017).
-
Dunlop, B. W. et al. Functional connectivity of the subcallosal cingulate cortex and differential outcomes to treatment with cognitive–behavioral therapy or antidepressant medication for major depressive disorder. Am. J. Psychiatry 174, 533–545 (2017).
-
Sani, O. G. et al. Mood variations decoded from multi-site intracranial human brain activity. Nat. Biotechnol. 36, 954–961 (2018).
-
Shanechi, M. M. Brain–machine interfaces from motor to mood. Nat. Neurosci. 22, 1554–1564 (2019).
-
Gray, J. P., Müller, V. I., Eickhoff, S. B. & Fox, P. T. Multimodal abnormalities of brain structure and function in major depressive disorder: a meta-analysis of neuroimaging studies. Am. J. Psychiatry 177, 422–434 (2020).
-
Bijanki, K. R. et al. Defining functional brain networks underlying obsessive–compulsive disorder (OCD) using treatment-induced neuroimaging changes: a systematic review of the literature. J. Neurol. Neurosurg. Psychiatry 92, 776–786 (2021).
-
Kohoutová, L. et al. Individual variability in brain representations of pain. Nat. Neurosci. 25, 749–759 (2022).
-
Xiao, J. et al. Decoding depression severity from intracranial neural activity. Biol. Psychiatry 94, 445–453 (2023).
-
Scangos, K. W., State, M. W., Miller, A. H., Baker, J. T. & Williams, L. M. New and emerging approaches to treat psychiatric disorders. Nat. Med. 29, 317–333 (2023).
-
Sheth, S. A. et al. Deep brain stimulation for depression informed by intracranial recordings. Biol. Psychiatry 92, 246–251 (2022).
-
Kirkby, L. A. et al. An amygdala–hippocampus subnetwork that encodes variation in human mood. Cell 175, 1688–1700.e14 (2018).
-
Basu, I. et al. Closed-loop enhancement and neural decoding of cognitive control in humans. Nat. Biomed. Eng. 7, 576–588 (2023).
-
Sendi, M. S. E. et al. Intraoperative neural signals predict rapid antidepressant effects of deep brain stimulation. Transl. Psychiatry 11, 551 (2021).
-
Shirvalkar, P. et al. First-in-human prediction of chronic pain state using intracranial neural biomarkers. Nat. Neurosci. 26, 1090–1099 (2023).
-
Nho, Y.-H. et al. Responsive deep brain stimulation guided by ventral striatal electrophysiology of obsession durably ameliorates compulsion. Neuron 112, 73–83.e4 (2024).
-
Kupfer, D. J., Frank, E. & Phillips, M. L. Major depressive disorder: new clinical, neurobiological, and treatment perspectives. Lancet 379, 1045–1055 (2012).
-
Boccard, S. G. J., Pereira, E. A. C. & Aziz, T. Z. Deep brain stimulation for chronic pain. J. Clin. Neurosci. 22, 1537–1543 (2015).
-
Shirvalkar, P., Veuthey, T. L., Dawes, H. E. & Chang, E. F. Closed-loop deep brain stimulation for refractory chronic pain. Front. Comput. Neurosci. 12, 18 (2018).
-
Lipsman, N. et al. Deep brain stimulation of the subcallosal cingulate for treatment-refractory anorexia nervosa: 1 year follow-up of an open-label trial. Lancet Psychiatry 4, 285–294 (2017).
-
Vloo, P. D. et al. Long-term follow-up of deep brain stimulation for anorexia nervosa. J. Neurol. Neurosurg. Psychiatry 92, 1135–1136 (2021).
-
Bina, R. W. & Langevin, J. P. Closed loop deep brain stimulation for PTSD, addiction, and disorders of affective facial interpretation: review and discussion of potential biomarkers and stimulation paradigms. Front. Neurosci. 12, 300 (2018).
-
Langevin, J.-P. et al. Deep brain stimulation of the basolateral amygdala for treatment-refractory posttraumatic stress disorder. Biol. Psychiatry 79, e82–e84 (2016).
-
de Hemptinne, C. et al. Prefrontal physiomarkers of anxiety and depression in Parkinson’s disease. Front. Neurosci. 15, 1337 (2021).
-
Fridgeirsson, E. A. et al. Patient specific intracranial neural signatures of obsessions and compulsions in the ventral striatum. J. Neural Eng. https://doi.org/10.1088/1741-2552/acbee1 (2023).
-
Montgomery, S. A. & Asberg, M. A new depression scale designed to be sensitive to change. Br. J. Psychiatry J. Ment. Sci. 134, 382–389 (1979).
-
Widge, A. S. et al. Patient-specific connectomic models correlate with, but do not reliably predict, outcomes in deep brain stimulation for obsessive–compulsive disorder. Neuropsychopharmacology 47, 965–972 (2022).
-
Hamilton, M. Development of a rating scale for primary depressive illness. Br. J. Soc. Clin. Psychol. 6, 278–296 (1967).
-
Nahum, M. et al. Immediate Mood Scaler: tracking symptoms of depression and anxiety using a novel mobile mood scale. JMIR MHealth UHealth 5, e44 (2017).
-
Goodman, W. K. et al. The Yale–Brown Obsessive Compulsive Scale: I. development, use, and reliability. Arch. Gen. Psychiatry 46, 1006–1011 (1989).
-
Provenza, N. R. et al. Long-term ecological assessment of intracranial electrophysiology synchronized to behavioral markers in obsessive–compulsive disorder. Nat. Med. 27, 2154–2164 (2021).
-
Gadot, R. et al. Tractography-based modeling explains treatment outcomes in patients undergoing deep brain stimulation for obsessive–compulsive disorder. Biol. Psychiatry https://doi.org/10.1016/j.biopsych.2023.01.017 (2023).
-
Haeffel, G. J. & Howard, G. S. Self-report: psychology’s four-letter word. Am. J. Psychol. 123, 181–188 (2010).
-
Dang, J., King, K. M. & Inzlicht, M. Why are self-report and behavioral measures weakly correlated? Trends Cogn. Sci. 24, 267–269 (2020).
-
Gibbons, R. D. et al. Development of a computerized adaptive test for depression. Arch. Gen. Psychiatry 69, 1104–1112 (2012).
-
Sani, S., Busnello, J., Kochanski, R., Cohen, Y. & Gibbons, R. D. High-frequency measurement of depressive severity in a patient treated for severe treatment-resistant depression with deep-brain stimulation. Transl. Psychiatry 7, e1207 (2017).
-
Ekman, P. & Friesen, W. V. Facial Action Coding System (Consulting Psychologists Press, 1977).
-
Tao, J. & Tan, T. in Affective Computing and Intelligent Interaction (eds Tao, J., Tan, T. & Picard, R. W.) 981–995 (Springer, 2005).
-
Weninger, F., Wöllmer, M. & Schuller, B. in Emotion Recognition (eds Konar, A. & Chakraborty, A.) 237–267 (John Wiley & Sons, 2015).
-
Sullivan, C. R. P., Olsen, S. & Widge, A. S. Deep brain stimulation for psychiatric disorders: from focal brain targets to cognitive networks. NeuroImage 225, 117515 (2021).
-
McTeague, L. M. et al. Identification of common neural circuit disruptions in cognitive control across psychiatric disorders. Am. J. Psychiatry 174, 676–685 (2017).
-
Yousefi, A. et al. Decoding hidden cognitive states from behavior and physiology using a Bayesian approach. Neural Comput. 31, 1751–1788 (2019).
-
Cuthbert, B. N. & Insel, T. R. Toward the future of psychiatric diagnosis: the seven pillars of RDoC. BMC Med. 11, 126 (2013).
-
Etkin, A. & Wager, T. D. Functional neuroimaging of anxiety: a meta-analysis of emotional processing in PTSD, social anxiety disorder, and specific phobia. Am. J. Psychiatry 164, 1476–1488 (2007).
-
Haber, S. N. et al. Circuits, networks, and neuropsychiatric disease: transitioning from anatomy to imaging. Biol. Psychiatry 87, 318–327 (2020).
-
Siddiqi, S. H. et al. Brain stimulation and brain lesions converge on common causal circuits in neuropsychiatric disease. Nat. Hum. Behav. 5, 1707–1716 (2021).
-
Mayberg, H. S. et al. Reciprocal limbic–cortical function and negative mood: converging PET findings in depression and normal sadness. Am. J. Psychiatry 156, 675–682 (1999).
-
Scangos, K. W., Makhoul, G. S., Sugrue, L. P., Chang, E. F. & Krystal, A. D. State-dependent responses to intracranial brain stimulation in a patient with depression. Nat. Med. 27, 229–231 (2021).
-
Ahmari, S. E. & Dougherty, D. D. Dissecting OCD circuits: from animal models to targeted treatments. Depress. Anxiety 32, 550–562 (2015).
-
Fettes, P., Schulze, L. & Downar, J. Cortico–striatal–thalamic loop circuits of the orbitofrontal cortex: promising therapeutic targets in psychiatric illness. Front. Syst. Neurosci. 11, 25 (2017).
-
Choi, K. S., Riva-Posse, P., Gross, R. E. & Mayberg, H. S. Mapping the ‘depression switch’ during intraoperative testing of subcallosal cingulate deep brain stimulation. JAMA Neurol. 72, 1252–1260 (2015).
-
Starr, P. A. Totally implantable bidirectional neural prostheses: a flexible platform for innovation in neuromodulation. Front. Neurosci. 12, 619 (2018).
-
Goyal, A. et al. The development of an implantable deep brain stimulation device with simultaneous chronic electrophysiological recording and stimulation in humans. Biosens. Bioelectron. 176, 112888 (2021).
-
Allawala, A. et al. A novel framework for network-targeted neuropsychiatric deep brain stimulation. Neurosurgery 89, E116–E120 (2021).
-
Scangos, K. W. et al. Pilot study of an intracranial electroencephalography biomarker of depressive symptoms in epilepsy. J. Neuropsychiatry Clin. Neurosci. 32, 185–190 (2020).
-
Herrington, T. M., Cheng, J. J. & Eskandar, E. N. Mechanisms of deep brain stimulation. J. Neurophysiol. 115, 19–38 (2016).
-
Ashkan, K., Rogers, P., Bergman, H. & Ughratdar, I. Insights into the mechanisms of deep brain stimulation. Nat. Rev. Neurol. 13, 548–554 (2017).
-
van Hartevelt, T. J. et al. Neural plasticity in human brain connectivity: the effects of long term deep brain stimulation of the subthalamic nucleus in Parkinson’s disease. PLoS ONE 9, e86496 (2014).
-
Merola, A. et al. New frontiers for deep brain stimulation: directionality, sensing technologies, remote programming, robotic stereotactic assistance, asleep procedures, and connectomics. Front. Neurol. 12, 1149 (2021).
-
Gilron, R. et al. Long-term wireless streaming of neural recordings for circuit discovery and adaptive stimulation in individuals with Parkinson’s disease. Nat. Biotechnol. 39, 1078–1085 (2021).
-
Larkin, H. D. Apple Watch Parkinson disease symptom monitor is cleared. JAMA 328, 416 (2022).
-
Frank, A. C., Li, R., Peterson, B. S. & Narayanan, S. S. Wearable and mobile technologies for the evaluation and treatment of obsessive–compulsive disorder: scoping review. JMIR Ment. Health 10, e45572 (2023).
-
Veerakumar, A. et al. Field potential 1/f activity in the subcallosal cingulate region as a candidate signal for monitoring deep brain stimulation for treatment-resistant depression. J. Neurophysiol. 122, 1023–1035 (2019).
-
Alagapan, S. et al. Cingulate dynamics track depression recovery with deep brain stimulation. Nature 622, 130–138 (2023).
-
Yang, Y., Sani, O. G., Chang, E. F. & Shanechi, M. M. Dynamic network modeling and dimensionality reduction for human ECoG activity. J. Neural Eng. 16, 056014 (2019).
-
Yang, Y., Connolly, A. T. & Shanechi, M. M. A control-theoretic system identification framework and a real-time closed-loop clinical simulation testbed for electrical brain stimulation. J. Neural Eng. 15, 066007 (2018).
-
Yang, Y. et al. Modelling and prediction of the dynamic responses of large-scale brain networks during direct electrical stimulation. Nat. Biomed. Eng. 5, 324–345 (2021).
-
Lozano, A. M. et al. Deep brain stimulation: current challenges and future directions. Nat. Rev. Neurol. 15, 148–160 (2019).
-
Grill, W. M., Norman, S. E. & Bellamkonda, R. V. Implanted neural interfaces: biochallenges and engineered solutions. Annu. Rev. Biomed. Eng. 11, 1–24 (2009).
-
Downey, J. E., Schwed, N., Chase, S. M., Schwartz, A. B. & Collinger, J. L. Intracortical recording stability in human brain–computer interface users. J. Neural Eng. 15, 046016 (2018).
-
Shenoy, K. V. & Carmena, J. M. Combining decoder design and neural adaptation in brain–machine interfaces. Neuron 84, 665–680 (2014).
-
Degenhart, A. D. et al. Stabilization of a brain–computer interface via the alignment of low-dimensional spaces of neural activity. Nat. Biomed. Eng. 4, 672–685 (2020).
-
Ahmadipour, P., Yang, Y., Chang, E. F. & Shanechi, M. M. Adaptive tracking of human ECoG network dynamics. J. Neural Eng. 18, 016011 (2021).
-
Yang, Y., Ahmadipour, P. & Shanechi, M. M. Adaptive latent state modeling of brain network dynamics with real-time learning rate optimization. J. Neural Eng. 18, 036013 (2021).
-
Dabagia, M., Kording, K. P. & Dyer, E. L. Aligning latent representations of neural activity. Nat. Biomed. Eng. 7, 337–343 (2023).
-
Hsieh, H.-L. & Shanechi, M. M. Optimizing the learning rate for adaptive estimation of neural encoding models. PLoS Comput. Biol. 14, e1006168 (2018).
-
Harper, R. & Southern, J. A Bayesian deep learning framework for end-to-end prediction of emotion from heartbeat. IEEE Trans. Affect. Comput. 13, 985–991 (2022).
-
Kim, J., Campbell, A. S., de Ávila, B. E.-F. & Wang, J. Wearable biosensors for healthcare monitoring. Nat. Biotechnol. 37, 389–406 (2019).
-
van Westen, M. et al. Optimizing deep brain stimulation parameters in obsessive–compulsive disorder. Neuromodulation 24, 307–315 (2021).
-
Gadot, R. et al. Efficacy of deep brain stimulation for treatment-resistant obsessive–compulsive disorder: systematic review and meta-analysis. J. Neurol. Neurosurg. Psychiatry 93, 1166–1173 (2022).
-
Sheth, S. A. & Mayberg, H. S. Deep brain stimulation for obsessive–compulsive disorder and depression. Annu. Rev. Neurosci. https://doi.org/10.1146/annurev-neuro-110122-110434 (2023).
-
Tsolaki, E., Espinoza, R. & Pouratian, N. Using probabilistic tractography to target the subcallosal cingulate cortex in patients with treatment resistant depression. Psychiatry Res. Neuroimaging 261, 72–74 (2017).
-
Liebrand, L. C. et al. Individual white matter bundle trajectories are associated with deep brain stimulation response in obsessive–compulsive disorder. Brain Stimulat. 12, 353–360 (2019).
-
Graat, I. et al. Tractography-based versus anatomical landmark-based targeting in vALIC deep brain stimulation for refractory obsessive–compulsive disorder. Mol. Psychiatry 27, 5206–5212 (2022).
-
Haber, S. N., Yendiki, A. & Jbabdi, S. Four deep brain stimulation targets for obsessive–compulsive disorder: are they different? Biol. Psychiatry 90, 667–677 (2021).
-
Stiso, J. et al. White matter network architecture guides direct electrical stimulation through optimal state transitions. Cell Rep. 28, 2554–2566.e7 (2019).
-
Horn, A. & Kühn, A. A. Lead-DBS: a toolbox for deep brain stimulation electrode localizations and visualizations. NeuroImage 107, 127–135 (2015).
-
Cagnan, H., Denison, T., McIntyre, C. & Brown, P. Emerging technologies for improved deep brain stimulation. Nat. Biotechnol. 37, 1024–1033 (2019).
-
Raymaekers, S., Luyten, L., Bervoets, C., Gabriëls, L. & Nuttin, B. Deep brain stimulation for treatment-resistant major depressive disorder: a comparison of two targets and long-term follow-up. Transl. Psychiatry 7, e1251 (2017).
-
Tyagi, H. et al. A randomized trial directly comparing ventral capsule and anteromedial subthalamic nucleus stimulation in obsessive–compulsive disorder: clinical and imaging evidence for dissociable effects. Biol. Psychiatry 85, 726–734 (2019).
-
Olsen, S. T. et al. Case report of dual-site neurostimulation and chronic recording of cortico–striatal circuitry in a patient with treatment refractory obsessive compulsive disorder. Front. Hum. Neurosci. 14, 569973 (2020).
-
Wu, H. et al. Local accumbens in vivo imaging during deep brain stimulation reveals a strategy-dependent amelioration of hedonic feeding. Proc. Natl Acad. Sci. USA 119, e2109269118 (2022).
-
Van Overschee, P. & De Moor, B. Subspace Identification for Linear Systems (Springer US, 1996).
-
McIntyre, C. C. & Hahn, P. J. Network perspectives on the mechanisms of deep brain stimulation. Neurobiol. Dis. 38, 329–337 (2010).
-
Santaniello, S. et al. Therapeutic mechanisms of high-frequency stimulation in Parkinson’s disease and neural restoration via loop-based reinforcement. Proc. Natl Acad. Sci. USA 112, E586–E595 (2015).
-
West, T. O. et al. Stimulating at the right time to recover network states in a model of the cortico–basal ganglia–thalamic circuit. PLoS Comput. Biol. 18, e1009887 (2022).
-
Feng, X. J., Shea-Brown, E., Greenwald, B., Kosut, R. & Rabitz, H. Optimal deep brain stimulation of the subthalamic nucleus — a computational study. J. Comput. Neurosci. 23, 265–282 (2007).
-
Stefanescu, R. A., Shivakeshavan, R. G. & Talathi, S. S. Computational models of epilepsy. Seizure 21, 748–759 (2012).
-
Sritharan, D. & Sarma, S. V. Fragility in dynamic networks: application to neural networks in the epileptic cortex. Neural Comput. 26, 2294–2327 (2014).
-
Brocker, D. T. et al. Optimized temporal pattern of brain stimulation designed by computational evolution. Sci. Transl. Med. 9, eaah3532 (2017).
-
Liu, J., Khalil, H. K. & Oweiss, K. G. Model-based analysis and control of a network of basal ganglia spiking neurons in the normal and parkinsonian states. J. Neural Eng. 8, 045002 (2011).
-
Santaniello, S., Fiengo, G., Glielmo, L. & Grill, W. M. Closed-loop control of deep brain stimulation: a simulation study. IEEE Trans. Neural Syst. Rehabil. Eng. 19, 15–24 (2011).
-
Millard, D. C., Wang, Q., Gollnick, C. A. & Stanley, G. B. System identification of the nonlinear dynamics in the thalamocortical circuit in response to patterned thalamic microstimulation in-vivo. J. Neural Eng. 10, 066011 (2013).
-
Bolus, M. F., Willats, A. A., Whitmire, C. J., Rozell, C. J. & Stanley, G. B. Design strategies for dynamic closed-loop optogenetic neurocontrol in vivo. J. Neural Eng. 15, 026011 (2018).
-
Ljung, L. System Identification: Theory for the User (Prentice-Hall, Inc., 1986).
-
Murphy, K. P. Machine Learning: A Probabilistic Perspective (MIT Press, 2013).
-
Bolus, M. F., Willats, A. A., Rozell, C. J. & Stanley, G. B. State-space optimal feedback control of optogenetically driven neural activity. J. Neural Eng. 18, 036006 (2021).
-
Basu, I. et al. Consistent linear and non-linear responses to invasive electrical brain stimulation across individuals and primate species with implanted electrodes. Brain Stimulat. 12, 877–892 (2019).
-
Bertsekas, D. P. Dynamic Programming and Optimal Control (Athena Scientific, 2005).
-
Abbaspourazad, H., Erturk, E., Pesaran, B. & Shanechi, M. M. Dynamical flexible inference of nonlinear latent factors and structures in neural population activity. Nat. Biomed. Eng. 8, 85–108 (2024).
-
Shanechi, M. M., Chemali, J. J., Liberman, M., Solt, K. & Brown, E. N. A brain–machine interface for control of medically-induced coma. PLoS Comput. Biol. 9, e1003284 (2013).
-
Yang, Y. & Shanechi, M. M. An adaptive and generalizable closed-loop system for control of medically induced coma and other states of anesthesia. J. Neural Eng. 13, 66019 (2016).
-
Yang, Y. et al. Developing a personalized closed-loop controller of medically-induced coma in a rodent model. J. Neural Eng. 16, 036022 (2019).
-
Hampson, R. E. et al. Developing a hippocampal neural prosthetic to facilitate human memory encoding and recall. J. Neural Eng. 15, 036014 (2018).
-
Lesort, T., Díaz-Rodríguez, N., Goudou, J.-F. & Filliat, D. State representation learning for control: an overview. Neural Netw. 108, 379–392 (2018).
-
Kremen, V. et al. Integrating brain implants with local and distributed computing devices: a next generation epilepsy management system. IEEE J. Transl. Eng. Health Med. https://doi.org/10.1109/JTEHM.2018.2869398 (2018).
-
Rouse, A. G. et al. A chronic generalized bi-directional brain–machine interface. J. Neural Eng. 8, 36018 (2011).
-
Stanslaski, S. et al. A chronically implantable neural coprocessor for investigating the treatment of neurological disorders. IEEE Trans. Biomed. Circuits Syst. 12, 1230–1245 (2018).
-
Sun, F. T. & Morrell, M. J. The RNS system: responsive cortical stimulation for the treatment of refractory partial epilepsy. Expert Rev. Med. Devices 11, 563–572 (2014).
-
Swann, N. C. et al. Chronic multisite brain recordings from a totally implantable bidirectional neural interface: experience in five patients with Parkinson’s disease. J. Neurosurg. 128, 605–616 (2018).
-
Krauss, J. K. et al. Technology of deep brain stimulation: current status and future directions. Nat. Rev. Neurol. 17, 75–87 (2021).
-
Arlotti, M. et al. A new implantable closed-loop clinical neural interface: first application in Parkinson’s disease. Front. Neurosci. 15, 763235 (2021).
-
Zhou, A., Johnson, B. C. & Muller, R. Toward true closed-loop neuromodulation: artifact-free recording during stimulation. Curr. Opin. Neurobiol. 50, 119–127 (2018).
-
Hashimoto, T., Elder, C. M. & Vitek, J. L. A template subtraction method for stimulus artifact removal in high-frequency deep brain stimulation. J. Neurosci. Methods 113, 181–186 (2002).
-
Rozgic, D. et al. A 0.338 cm3, artifact-free, 64-contact neuromodulation platform for simultaneous stimulation and sensing. IEEE Trans. Biomed. Circuits Syst. 13, 38–55 (2019).
-
Zhou, A. et al. A wireless and artefact-free 128-channel neuromodulation device for closed-loop stimulation and recording in non-human primates. Nat. Biomed. Eng. 3, 15–26 (2019).
-
Kohl, S. et al. Deep brain stimulation for treatment-refractory obsessive compulsive disorder: a systematic review. BMC Psychiatry 14, 214 (2014).
-
An, H. et al. A power-efficient brain–machine interface system with a sub-mw feature extraction and decoding ASIC demonstrated in nonhuman primates. IEEE Trans. Biomed. Circuits Syst. 16, 395–408 (2022).
-
Shoaran, M., Haghi, B. A., Taghavi M., Farivar M. & Emami-Neyestanak A. Energy-efficient classification for resource-constrained biomedical applications. IEEE J. Emerg. Sel. Top. Circuits Syst. 8, 693–707 (2018).
-
Hurwitz, C., Kudryashova, N., Onken, A. & Hennig, M. H. Building population models for large-scale neural recordings: opportunities and pitfalls. Curr. Opin. Neurobiol. 70, 64–73 (2021).
-
Sarchiapone, M. et al. The association between electrodermal activity (EDA), depression and suicidal behaviour: a systematic review and narrative synthesis. BMC Psychiatry 18, 22 (2018).
-
Wickramasuriya, D. S., Amin, Md R. & Faghih, R. T. Skin conductance as a viable alternative for closing the deep brain stimulation loop in neuropsychiatric disorders. Front. Neurosci. 13, 780 (2019).
-
van Eck, M., Berkhof, H., Nicolson, N. & Sulon, J. The effects of perceived stress, traits, mood states, and stressful daily events on salivary cortisol. Psychosom. Med. 58, 447–458 (1996).
-
Young, A. H. Cortisol in mood disorders. Stress 7, 205–208 (2004).
-
Chesnut, M. et al. Stress markers for mental states and biotypes of depression and anxiety: a scoping review and preliminary illustrative analysis. Chronic Stress 5, 24705470211000336 (2021).
-
Topalovic, U. et al. Wireless programmable recording and stimulation of deep brain activity in freely moving humans. Neuron 108, 322–334.e9 (2020).
-
Nason, S. R. et al. A low-power band of neuronal spiking activity dominated by local single units improves the performance of brain–machine interfaces. Nat. Biomed. Eng. 4, 973–983 (2020).
-
Paulk, A. C. et al. Large-scale neural recordings with single neuron resolution using Neuropixels probes in human cortex. Nat. Neurosci. 25, 252–263 (2022).
-
Hsieh, H. L., Wong, Y. T., Pesaran, B. & Shanechi, M. M. Multiscale modeling and decoding algorithms for spike-field activity. J. Neural Eng. 16, 016018 (2019).
-
Topalovic, U. et al. A wearable platform for closed-loop stimulation and recording of single-neuron and local field potential activity in freely moving humans. Nat. Neurosci. 26, 517–527 (2023).
-
Abbaspourazad, H., Choudhury, M., Wong, Y. T., Pesaran, B. & Shanechi, M. M. Multiscale low-dimensional motor cortical state dynamics predict naturalistic reach-and-grasp behavior. Nat. Commun. 12, 607 (2021).
-
Abbaspourazad, H., Hsieh, H. & Shanechi, M. M. A multiscale dynamical modeling and identification framework for spike-field activity. IEEE Trans. Neural Syst. Rehabil. Eng. 27, 1128–1138 (2019).
-
Wang, C. & Shanechi, M. M. Estimating multiscale direct causality graphs in neural spike-field networks. IEEE Trans. Neural Syst. Rehabil. Eng. 27, 857–866 (2019).
-
Sani, O. G., Abbaspourazad, H., Wong, Y. T., Pesaran, B. & Shanechi, M. M. Modeling behaviorally relevant neural dynamics enabled by preferential subspace identification. Nat. Neurosci. 24, 140–149 (2021).
-
Song, C. Y., Hsieh, H.-L., Pesaran, B. & Shanechi, M. M. Modeling and inference methods for switching regime-dependent dynamical systems with multiscale neural observations. J. Neural Eng. 19, 066019 (2022).
-
Stangl, M., Maoz, S. L. & Suthana, N. Mobile cognition: imaging the human brain in the ‘real world’. Nat. Rev. Neurosci. 24, 347–362 (2023).
-
Brown, T. et al. Controlling our brains — a case study on the implications of brain–computer interface-triggered deep brain stimulation for essential tremor. Brain Comput. Interfaces 3, 165–170 (2016).
-
Klein, E. et al. Brain–computer interface-based control of closed-loop brain stimulation: attitudes and ethical considerations. Brain Comput. Interfaces 3, 140–148 (2016).
-
Yuste, R. et al. Four ethical priorities for neurotechnologies and AI. Nature 551, 159–163 (2017).
-
Greenberg, B. D. et al. Deep brain stimulation of the ventral internal capsule/ventral striatum for obsessive–compulsive disorder: worldwide experience. Mol. Psychiatry 15, 64–79 (2010).
-
Hamani, C. & Temel, Y. Deep brain stimulation for psychiatric disease: contributions and validity of animal models. Sci. Transl. Med. 4, 142rv8 (2012).
-
Deisseroth, K. Optogenetics. Nat. Methods 8, 26–29 (2011).
-
Machado, T. A., Kauvar, I. V. & Deisseroth, K. Multiregion neuronal activity: the forest and the trees. Nat. Rev. Neurosci. 23, 683–704 (2022).
-
Tye, K. M. et al. Dopamine neurons modulate neural encoding and expression of depression-related behaviour. Nature 493, 537–541 (2013).
-
Namburi, P. et al. A circuit mechanism for differentiating positive and negative associations. Nature 520, 675–678 (2015).
-
Calhoon, G. G. & Tye, K. M. Resolving the neural circuits of anxiety. Nat. Neurosci. 18, 1394–1404 (2015).
-
Janssen, M. L. F. et al. Cortico-subthalamic inputs from the motor, limbic, and associative areas in normal and dopamine-depleted rats are not fully segregated. Brain Struct. Funct. 222, 2473–2485 (2017).
-
Hultman, R. et al. Brain-wide electrical spatiotemporal dynamics encode depression vulnerability. Cell 173, 166–180.e14 (2018).
-
Mague, S. D. et al. Brain-wide electrical dynamics encode individual appetitive social behavior. Neuron 110, 1728–1741.e7 (2022).
-
Wu, H. et al. Closing the loop on impulsivity via nucleus accumbens delta-band activity in mice and man. Proc. Natl Acad. Sci. USA 115, 192–197 (2018).
-
Hamani, C. et al. Deep brain stimulation reverses anhedonic-like behavior in a chronic model of depression: role of serotonin and brain derived neurotrophic factor. Biol. Psychiatry 71, 30–35 (2012).
-
Lim, L. W. et al. Electrical stimulation alleviates depressive-like behaviors of rats: investigation of brain targets and potential mechanisms. Transl. Psychiatry 5, e535 (2015).
-
Rappel, P. et al. Subthalamic theta activity: a novel human subcortical biomarker for obsessive compulsive disorder. Transl. Psychiatry 8, 118 (2018).
-
Miller, K. J., Prieto, T., Williams, N. R. & Halpern, C. H. Case studies in neuroscience: the electrophysiology of a human obsession in nucleus accumbens. J. Neurophysiol. 121, 2336–2340 (2019).
-
Ramasubbu, R., Anderson, S., Haffenden, A., Chavda, S. & Kiss, Z. H. T. Double-blind optimization of subcallosal cingulate deep brain stimulation for treatment-resistant depression: a pilot study. J. Psychiatry Neurosci. 38, 325–332 (2013).
-
Zhang, C. et al. Bilateral habenula deep brain stimulation for treatment-resistant depression: clinical findings and electrophysiological features. Transl. Psychiatry 12, 52 (2022).
-
Fenoy, A. J. et al. Deep brain stimulation of the medial forebrain bundle: distinctive responses in resistant depression. J. Affect. Disord. 203, 143–151 (2016).
-
Coenen, V. A. et al. Superolateral medial forebrain bundle deep brain stimulation in major depression: a gateway trial. Neuropsychopharmacology 44, 1224–1232 (2019).
-
Gálvez, J. F. et al. The medial forebrain bundle as a deep brain stimulation target for treatment resistant depression: a review of published data. Prog. Neuropsychopharmacol. Biol. Psychiatry 58, 59–70 (2015).
-
Goodman, W. K. et al. Deep brain stimulation for intractable obsessive compulsive disorder: pilot study using a blinded, staggered-onset design. Biol. Psychiatry 67, 535–542 (2010).
-
Bewernick, B. H., Kayser, S., Sturm, V. & Schlaepfer, T. E. Long-term effects of nucleus accumbens deep brain stimulation in treatment-resistant depression: evidence for sustained efficacy. Neuropsychopharmacology 37, 1975–1985 (2012).
-
Barcia, J. A. et al. Personalized striatal targets for deep brain stimulation in obsessive–compulsive disorder. Brain Stimulat. 12, 724–734 (2019).
-
Bergfeld, I. O. et al. Deep brain stimulation of the ventral anterior limb of the internal capsule for treatment-resistant depression: a randomized clinical trial. JAMA Psychiatry 73, 456–464 (2016).
-
Abelson, J. L. et al. Deep brain stimulation for refractory obsessive–compulsive disorder. Biol. Psychiatry 57, 510–516 (2005).
-
Mosley, P. E. et al. A randomised, double-blind, sham-controlled trial of deep brain stimulation of the bed nucleus of the stria terminalis for treatment-resistant obsessive–compulsive disorder. Transl. Psychiatry 11, 190 (2021).
-
Jiménez, F. et al. A patient with a resistant major depression disorder treated with deep brain stimulation in the inferior thalamic peduncle. Neurosurgery 57, 585–593 (2005).
-
Jiménez, F. et al. Neuromodulation of the inferior thalamic peduncle for major depression and obsessive compulsive disorder. Acta Neurochir. Suppl. 97, 393–398 (2007).
-
Roweis, S. & Ghahramani, Z. A unifying review of linear Gaussian models. Neural Comput. 11, 305–345 (1999).
-
Cunningham, J. P. & Yu, B. M. Dimensionality reduction for large-scale neural recordings. Nat. Neurosci. 17, 1500–1509 (2014).
-
Vyas, S., Golub, M. D., Sussillo, D. & Shenoy, K. V. Computation through neural population dynamics. Annu. Rev. Neurosci. 43, 249–275 (2020).
-
Vahidi, P., Sani, O. G. & Shanechi, M. M. Modeling and dissociation of intrinsic and input-driven neural population dynamics underlying behavior. Proc. Natl Acad. Sci. USA 121, e2212887121 (2024).
-
Camacho, E. F. & Bordons, C. Model Predictive Control (Springer, 2007).
-
Nozari, E. et al. Macroscopic resting-state brain dynamics are best described by linear models. Nat. Biomed. Eng. 8, 68–84 (2024).
Acknowledgements
The authors thank H. C. Jo, O. G. Sani, T. Jani and N. Sadras in the Shanechi laboratory for helpful feedback. This work was partly supported by the US National Institutes of Health grants R01MH123770 and R61MH135407, the One Mind Rising Star Award, and the Foundation for OCD Research.
Author information
Authors and Affiliations
Contributions
L.L.O. and M.M.S. developed the content and wrote the manuscript.
Corresponding author
Ethics declarations
Competing interests
M.M.S. is an inventor on University of Southern California’s patents or patent applications related to decoding and closed-loop control approaches, and is a consultant for Paradromics Inc. L.L.O. declares no competing interests.
Peer review
Peer review information
Nature Reviews Bioengineering thanks David Borton, Andreas Horn and Jean-Philippe Langevin for their contribution to the peer review of this work.
Additional information
Publisher’s note Springer Nature remains neutral with regard to jurisdictional claims in published maps and institutional affiliations.
Rights and permissions
Springer Nature or its licensor (e.g. a society or other partner) holds exclusive rights to this article under a publishing agreement with the author(s) or other rightsholder(s); author self-archiving of the accepted manuscript version of this article is solely governed by the terms of such publishing agreement and applicable law.
About this article
Cite this article
Oganesian, L.L., Shanechi, M.M. Brain–computer interfaces for neuropsychiatric disorders. Nat Rev Bioeng (2024). https://ift.tt/HhcsSuT
-
Accepted:
-
Published:
-
DOI: https://ift.tt/HhcsSuT
"interface" - Google News
June 03, 2024 at 07:50PM
https://ift.tt/UzEZ8Jt
Brain–computer interfaces for neuropsychiatric disorders - Nature.com
"interface" - Google News
https://ift.tt/bXpDzBS
https://ift.tt/YlGdm96
Bagikan Berita Ini
0 Response to "Brain–computer interfaces for neuropsychiatric disorders - Nature.com"
Post a Comment